In the realm of computing technology, there is nothing quite as powerful and complex as the human brain. With its 86 billion neurons and up to a quadrillion synapses, the brain has unparalleled capabilities for processing information. Unlike traditional computing devices with physically separated units, the brain’s efficiency lies in its ability to serve as both a processor and memory device. Recognizing the potential of harnessing the brain’s power, researchers have been striving to create more brain-like computing systems.
Efforts to mimic the brain’s activity in artificial systems have been ongoing, but progress has been limited. Even one of the most powerful supercomputers in the world, Riken’s K Computer, struggled to simulate just a fraction of the brain’s activity. With its 82,944 processors and a petabyte of main memory, it took 40 minutes to simulate just one second of the activity of 1.73 billion neurons connected by 10.4 trillion synapses. This represented only one to two percent of the brain’s capacity.
In recent years, scientists and engineers have delved into the realm of neuromorphic computing, which aims to replicate the brain’s structure and functionality. By designing hardware and algorithms that mimic the brain, researchers hope to overcome the limitations of traditional computing and improve energy efficiency. However, despite significant progress, neuromorphic computing still poses challenges, such as high energy consumption and time-consuming training of artificial neural networks.
Brainoware: The Integration of Human Brain Tissue and Electronics
Taking a bold step towards creating a more brain-like computing architecture, a team of researchers led by engineer Feng Guo from Indiana University Bloomington has developed an innovative approach called Brainoware. This groundbreaking system integrates real human brain tissue with electronics, bridging the gap between biology and technology. Brain organoids are three-dimensional mini-brains made from human pluripotent stem cells. Guo and his team have made a hybrid biocomputer that can do complicated things like recognizing speech and predicting nonlinear equations.
Brain organoids offer a unique opportunity to study the development and functionality of the brain without invasive procedures. These mini-brains are created by coaxing human pluripotent stem cells to differentiate into various types of brain cells that self-organize into three-dimensional structures resembling the human brain. While they lack consciousness and thought, brain organoids provide valuable insights into neural development and function.
Brainoware consists of brain organoids connected to an array of high-density microelectrodes. This connection allows for electrical stimulation to transport information into the organoid, which acts as a reservoir for processing that information. The output of the organoid is then conveyed through neural activity. To complete the system, traditional computer hardware is used for the input and output layers. These layers are trained to interact with the organoid, with the output layer reading the neural data and making classifications or predictions based on the input.
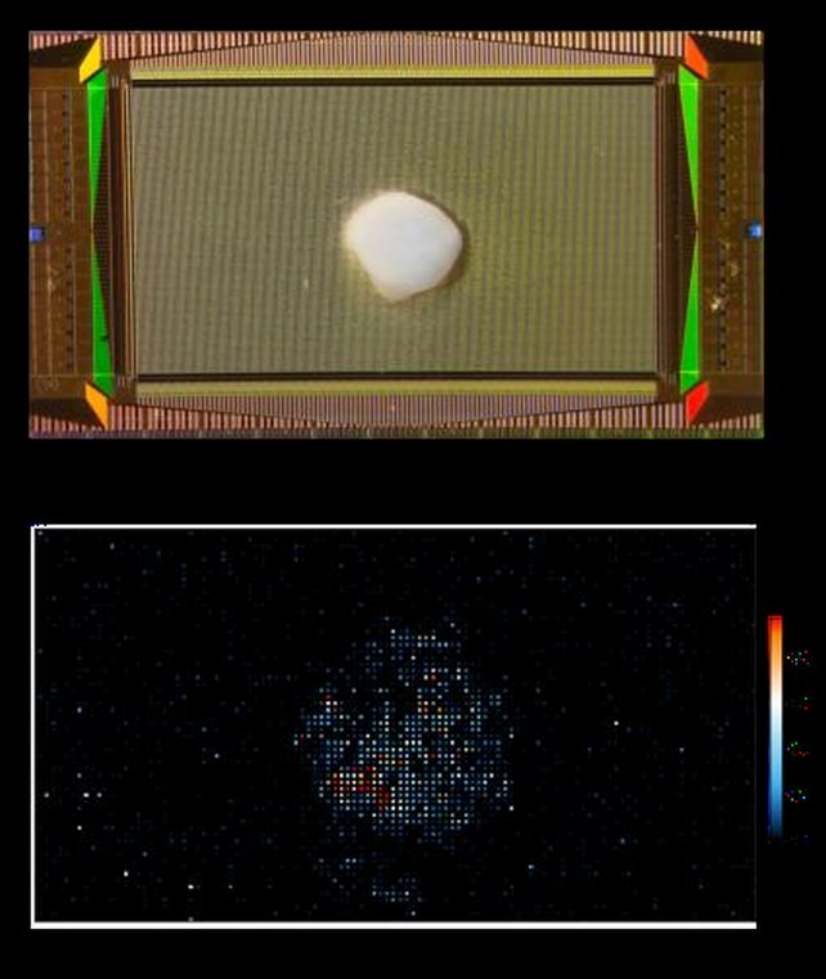
The Power of Brainoware: Speech Recognition
To demonstrate the capabilities of Brainoware, the researchers conducted experiments in speech recognition. They provided the system with 240 audio clips of eight male speakers producing Japanese vowel sounds and tasked it with identifying a specific individual’s voice. After just two days of training, Brainoware achieved an impressive accuracy rate of 78 percent. While slightly less accurate than a pure hardware computer running on artificial intelligence, this is a significant milestone in the development of a new kind of computing system.
In addition to speech recognition, Brainoware showcased its ability to predict complex mathematical systems. The researchers presented the system with a Hénon map, a nonlinear dynamic system known for its chaotic behavior. After four days of unsupervised learning, Brainoware successfully predicted the map with greater accuracy than an artificial neural network without a long short-term memory unit. Although it fell slightly short of the accuracy that neural networks with extensive training were able to achieve, Brainoware achieved these results in a fraction of the training time.
Brainoware offers several advantages over traditional computing systems. Its integration of human brain tissue allows for high plasticity and adaptability, enabling the system to change and reorganize in response to electrical stimulation. This flexibility highlights Brainoware’s potential for adaptive reservoir computing and its ability to process information more efficiently than conventional hardware.
However, there are still challenges to overcome. Keeping the brain organoids alive and healthy remains a significant limitation. Additionally, the power consumption of the peripheral equipment needs to be addressed. Ethical considerations are also essential as the sophistication of organoid systems increases. Researchers must address the neuroethical issues surrounding biocomputing systems that incorporate human neural tissue.
The Future of Brainoware and Biocomputing
While general biocomputing systems may still be decades away, the development of Brainoware has generated foundational insights into learning mechanisms, neural development, and the cognitive implications of neurodegenerative diseases. Furthermore, Brainoware has the potential to contribute to the development of preclinical models of cognitive impairment for testing new therapeutics.
With its integration of human brain tissue and electronics, Brainoware represents a significant step towards creating more brain-like computing systems. By harnessing the power and complexity of the human brain, researchers are paving the way for a new era of computing that holds promise for both technological advancement and a deeper understanding of the mysteries of the human mind.
References:
Cai, H., Ao, Z., Tian, C. et al. Brain organoid reservoir computing for artificial intelligence. Nat Electron (2023). DOI: 10.1038/s41928-023-01069-w
Smirnova, L., Caffo, B. & Johnson, E.C. Reservoir computing with brain organoids. Nat Electron (2023). DOI: 10.1038/s41928-023-01096-7
[…] Read more […]